The use of automated AI algorithms in organic chemistry and synthesis has been a considerable boon. While the potential applications for AI are vast in this space, only a few have been realized to a working extent. At present, AI and its various facets from conventional modeling to complex GANs (general adversarial networks) and VAEs (Variational Auto Encoders) have been used for the purpose of prediction, process control, identification, and synthesis.
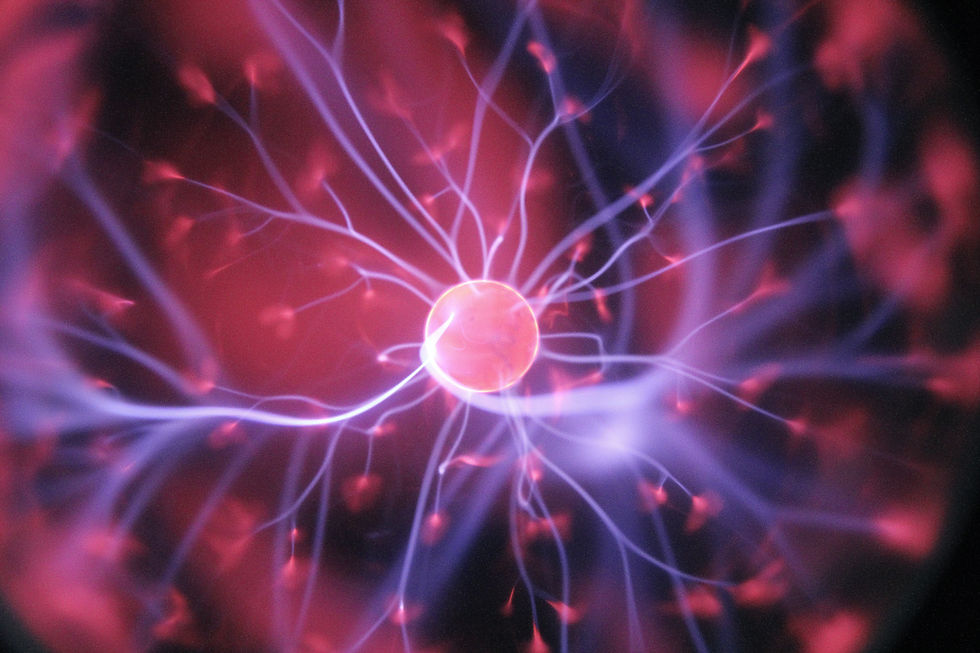
Computational algorithms aimed at the subject of organic synthesis are a great deal of interest in the coding community and represent another application for quantum computing.
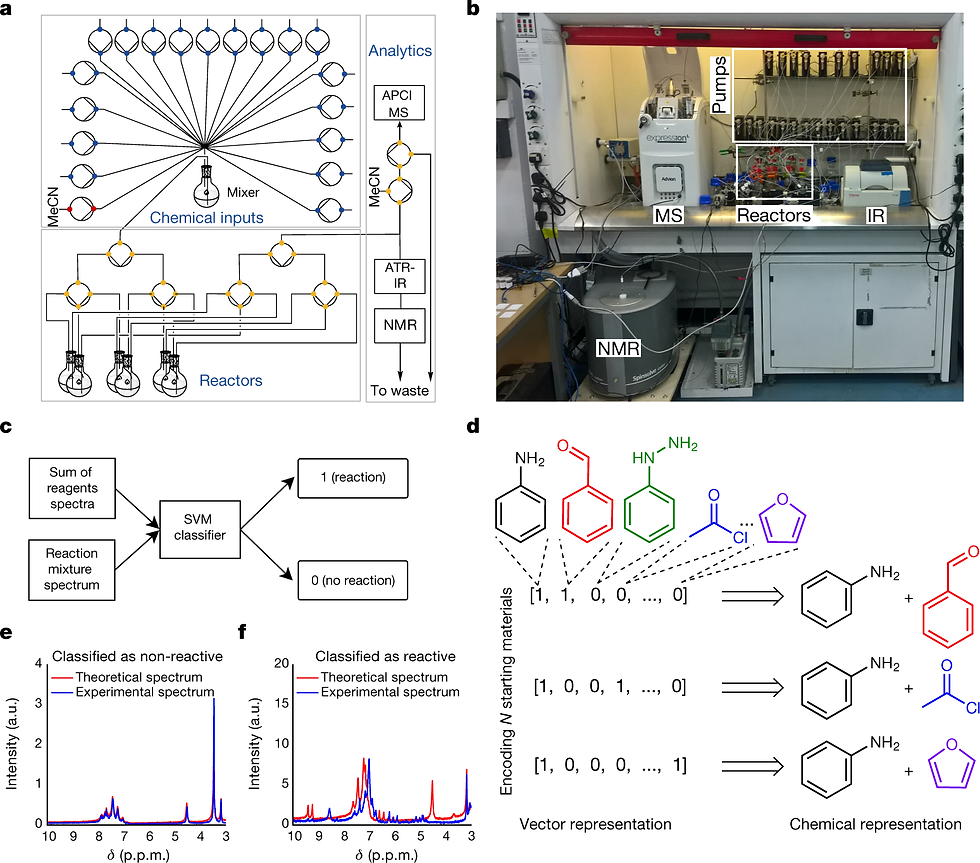
Source:- Organic Synthesis Setup
Retrosynthesis and AI algorithms
Computational retrosynthetic analysis tools are the more conventional example of algorithms that can potentially greatly assist chemists in designing synthetic routes to novel molecules. Some applications for these include drug discovery, medicinal chemistry, materials science, and natural product synthesis.
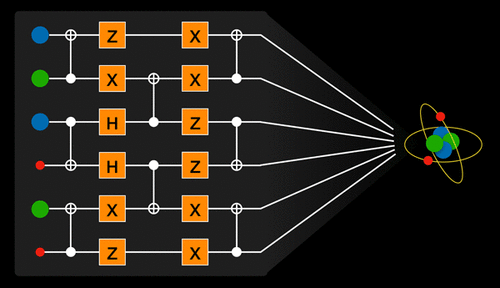
Such developments have been in effect since the 1960s when chemists recognized the promise of modern computing in assisting organic synthesis analyses. In part this is due to these approaches being applicable to only relatively simple target molecules for which expert chemists could readily deduce synthetic plans without assistance. Some of the areas where quantum computing could find greater relevance include:-
Algorithms:- Reaction rules are either manually encoded by human experts or automatically derived from a reaction database. The key drawback of such rule-based expert systems is that they generally cannot make accurate predictions outside of their knowledge base which can be amended using quantum computing.
The second class of algorithms uses principles of physical chemistry to predict energy barriers of a reaction based on the first principles. Quantum computing algorithms can be used in this context to generalize novel molecules and reaction types which are often prohibitively expensive to perform for full synthetic planning problems.
Quantum computing can also be used to deal with rule-based and physical chemistry approaches by making predictions that generalize better than those of rule-based approaches at a computational cost that is much less than those of physical chemistry approaches.
What The Future Appears To Be
As recently as 2020, the shift in data collection and algorithms to a centralized point has been the focus of the industry. As more and more algorithms specific to reactions and reaction mechanisms are designed, packages that encompass all the various types of reaction topologies will become a reality, allowing users to design experiments for all-purpose property predictions. Extensions to automated machine learning methods are another space of research that has garnered a great deal of interest.
It should be noted that more modern deep learning approaches combine a rule-based expert system with a feedforward neural network (NN) component that performs candidate ranking for reactions and properties. This may take a longer period to be replaced with more standardized quantum computing algorithms.
Tune into the next articles from the CybraneX team as we explore additional functionalities for quantum computing and chemical reactions for property predictions.
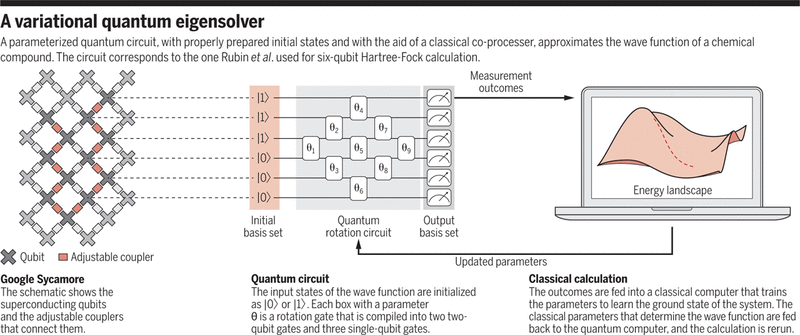
Source:- Eigensolvers for reaction chemistry
Comments